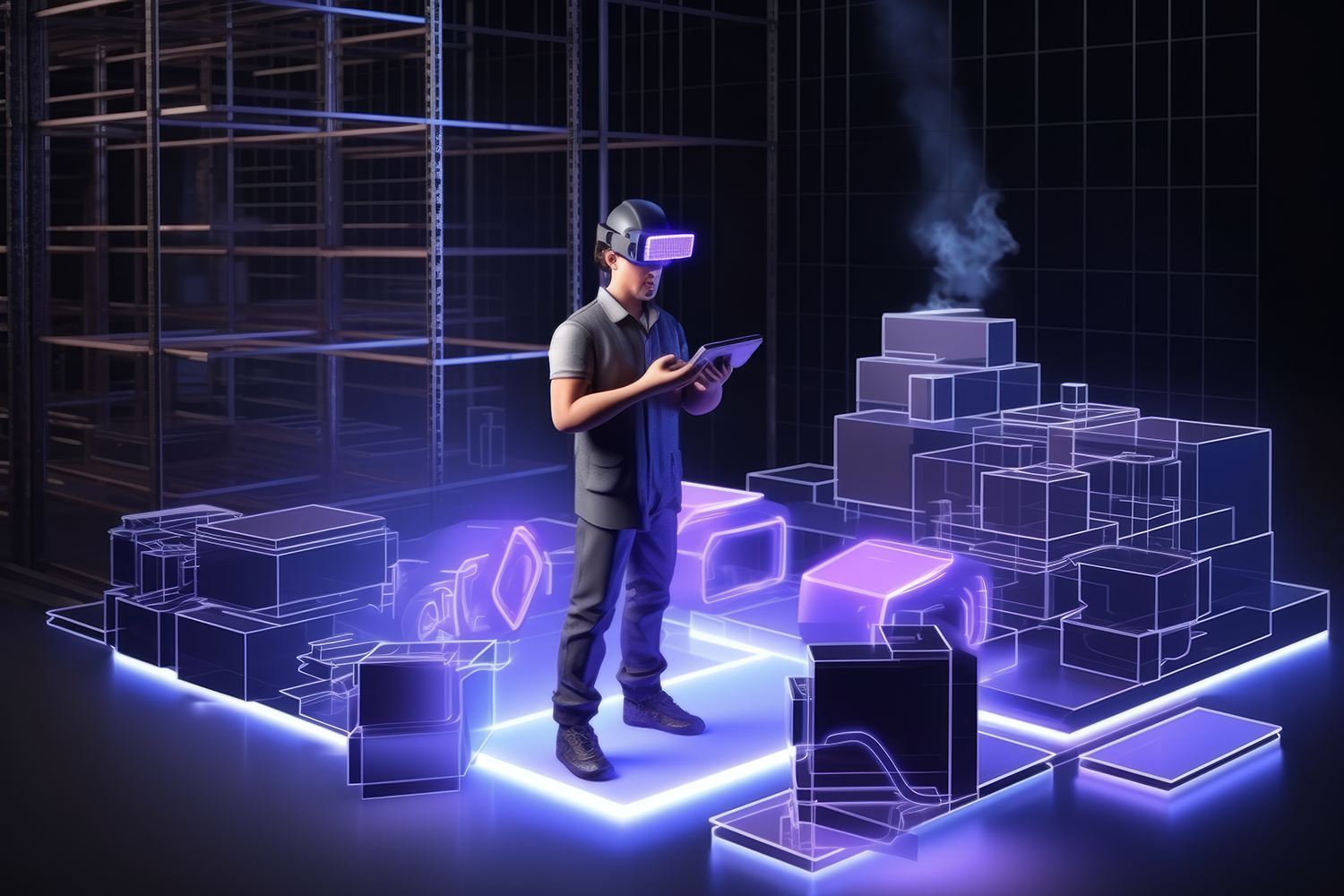
Transforming Maintenance with Machine Learning: The Future of Industry
Explore how machine learning is revolutionizing maintenance across industries and shaping the future of operations.
Machine learning (ML) is poised to disrupt maintenance strategies, making them smarter, more efficient, and predictive. In this blog, we explore how machine learning is changing the game and what businesses can expect in the future.
Machine Learning in Maintenance: An Overview
Machine learning is increasingly being integrated into maintenance processes across industries, offering a more predictive, data-driven approach to operational efficiency.
Machine learning algorithms can analyze historical data and predict when equipment will fail, enabling timely repairs and reducing downtime.
Data-Driven Decision Making
ML uses vast amounts of data to identify patterns and trends, empowering maintenance teams to make data-driven decisions and optimize resources.
Real-Time Monitoring
Continuous monitoring powered by machine learning enables the detection of anomalies in equipment behavior, helping prevent breakdowns before they happen.
Anomaly Detection
By identifying unusual patterns or behaviors, ML can detect faults and potential failures, reducing the need for manual inspections and reactive maintenance.
Self-Learning Systems
ML systems can evolve over time, improving their accuracy and predictions as more data is processed, creating a continuously optimizing maintenance environment.
How Machine Learning is Revolutionizing Maintenance Across Industries
Machine learning is making a significant impact in a variety of sectors, changing how businesses approach maintenance, repair, and operations.
In manufacturing, ML helps predict machine failures, leading to fewer disruptions, reduced operational costs, and increased productivity.
Energy Sector
Machine learning aids in the maintenance of power plants, optimizing turbine performance, predicting faults, and reducing energy production downtime.
Transportation & Logistics
In the transportation industry, ML is used to monitor vehicle fleets, predict when parts need replacement, and streamline maintenance schedules.
Aerospace
In aerospace, ML helps with predictive maintenance on aircraft, ensuring safety and reducing the frequency of unexpected maintenance checks.
Healthcare
ML is transforming the maintenance of medical devices and equipment, ensuring timely repairs and minimizing equipment failure in critical care environments.
What’s Next for Machine Learning in Maintenance?
The integration of machine learning into maintenance is an evolving field, and several key trends are shaping its future.
As ML models become more sophisticated, predictive analytics will improve, offering more precise forecasts and early detection of equipment failures.
Integration with IoT
Machine learning will increasingly be integrated with Internet of Things (IoT) devices, enabling more detailed and real-time data collection for better maintenance decisions.
Automated Repairs and Robots
The future of machine learning in maintenance will see increased use of robots and automation for performing maintenance tasks with minimal human intervention.
Augmented Reality (AR) and Machine Learning Integration
AR will combine with ML to provide real-time maintenance support, such as overlaying repair instructions on equipment, which will be enhanced by predictive data.
How Machine Learning in Maintenance Drives Business Success
Implementing machine learning in maintenance processes can provide several business benefits, improving overall operations and reducing costs.
With predictive maintenance and real-time monitoring, businesses can prevent unplanned downtime, leading to higher productivity and fewer interruptions in operations.
Cost Savings
By shifting from reactive to proactive maintenance, companies can reduce repair costs, extend equipment lifespan, and avoid expensive emergency fixes.
Improved Equipment Lifespan
ML-driven insights allow businesses to maintain equipment more effectively, preventing wear and tear and prolonging the lifecycle of costly machinery.
Optimized Resource Allocation
ML enables smarter allocation of maintenance resources by forecasting when and where maintenance will be needed, improving scheduling and efficiency.
Higher Safety Standards
By identifying potential issues before they escalate, ML can contribute to a safer working environment, preventing accidents and ensuring compliance with safety regulations.
Implementing Machine Learning in Maintenance: Key Challenges
While the benefits are clear, integrating machine learning into maintenance systems comes with certain challenges that businesses need to be aware of.
Machine learning relies on high-quality, accurate data. Gathering and cleaning data from various sources can be time-consuming and costly.
Integration with Existing Systems
Integrating machine learning algorithms with legacy systems or current maintenance software can be complex and require substantial infrastructure changes.
Initial Investment
The upfront cost of implementing machine learning solutions, including hardware, software, and training, can be significant.
Skillset Requirements
Developing and managing machine learning models requires specialized knowledge, making it essential to either upskill existing staff or hire experts in the field.
Resistance to Change
Employees and stakeholders may resist the shift toward automated, data-driven decision-making, especially if it threatens established workflows or job functions.
Maximizing the Potential of Machine Learning in Maintenance
To ensure the successful implementation of machine learning in maintenance, follow these best practices and tips.
Test machine learning solutions on a small scale before expanding, ensuring they work effectively with your systems and infrastructure.
Focus on High-Impact Areas First
Begin by applying machine learning to critical equipment or areas with the highest downtime to see the most immediate return on investment.
Ensure Data Quality
Prioritize clean, accurate data collection and processing to ensure your machine learning models are trained with reliable information.
Integrate with IoT Devices
Use IoT devices to collect real-time data, providing machine learning models with the information they need to make accurate predictions.
Leverage Cloud Computing
Utilize cloud platforms for scalable data storage, processing, and machine learning model training, avoiding costly on-premises infrastructure.
Monitor and Adjust Algorithms Regularly
Machine learning models require continuous monitoring and fine-tuning to ensure they remain accurate and effective over time.
Train Staff and Foster Collaboration
Invest in training your maintenance team to use ML-driven insights and encourage collaboration between data scientists and maintenance personnel.
Key Terms to Know
Using data-driven algorithms to predict equipment failure and schedule timely maintenance to avoid downtime.
Machine Learning (ML)
A subset of AI where algorithms learn from data and improve their performance over time without explicit programming.
Anomaly Detection
Identifying deviations from normal behavior in equipment, which may indicate a fault or potential failure.
Internet of Things (IoT)
A network of interconnected devices that collect and exchange data to improve decision-making.
Data Mining
The process of extracting valuable information from large datasets to identify patterns or trends.
Model Training
The process of teaching a machine learning model by feeding it data and adjusting its parameters to improve accuracy.
Artificial Intelligence (AI)
A broad field of computer science focused on creating systems that can perform tasks that typically require human intelligence.
Automation
Using technology to perform tasks with minimal human intervention, improving efficiency and reducing human error.
Cloud Computing
Using remote servers to store, manage, and process data, enabling scalable machine learning applications.
Real-Time Monitoring
Continuously tracking equipment or systems, enabling immediate response to any anomalies or issues detected.
What’s Next for Machine Learning in Maintenance?
The future of machine learning in maintenance holds exciting prospects, offering even more advanced capabilities for businesses.
Expect a deeper integration of AI alongside ML to create more sophisticated maintenance solutions that learn from diverse data sources.
More Autonomous Systems
As machine learning algorithms improve, we’ll see more autonomous systems capable of performing self-maintenance and repairs.
Enhanced Predictive Models
Machine learning models will become even more accurate, forecasting potential failures with pinpoint precision and enabling smarter decision-making.
Seamless IoT Integration
The combination of IoT and machine learning will result in more connected, efficient maintenance operations, providing real-time insights for all stakeholders.
Wider Industry Adoption
Machine learning will expand to more industries, with small and medium businesses increasingly adopting predictive maintenance solutions for cost savings.
Machine Learning: The Future of Maintenance in Industry
Machine learning is revolutionizing the maintenance sector by enhancing predictive capabilities, reducing costs, and increasing operational efficiency. As businesses continue to adopt ML-driven solutions, they will see long-term benefits in equipment management, safety, and overall productivity, positioning themselves for future success in a rapidly evolving industry.